The Future of Emotion Recognition: AI That Understands Customer Feelings
Estimated Reading Time: 7 minutes
Key Takeaways
- Emotion recognition AI is transforming how businesses understand and respond to customer sentiment
- Modern emotion AI can detect subtle emotional cues from text, voice, and facial expressions
- Ethical considerations around privacy and consent are crucial for responsible implementation
- The future of customer support includes emotionally intelligent AI systems that can respond with appropriate empathy
- Combining multimodal emotion analysis with conversational AI creates more human-like customer experiences
Table of Contents
- What is Emotion Recognition AI?
- The Science Behind Emotion Detection
- Current Applications in Customer Support
- Ethical Considerations and Privacy
- The Future of Emotionally Intelligent AI
- Integrating Emotion AI into Your Business
- Frequently Asked Questions
What is Emotion Recognition AI?
Emotion Recognition AI, also known as affective computing, is a branch of artificial intelligence that focuses on identifying, analyzing, and responding to human emotions. These systems use various inputs to detect emotional states, including text analysis, voice tone assessment, and facial expression recognition.
Unlike traditional AI systems that focus purely on functional responses, emotion recognition technology aims to understand the customer on a deeper level – identifying not just what they’re saying, but how they’re feeling about it.
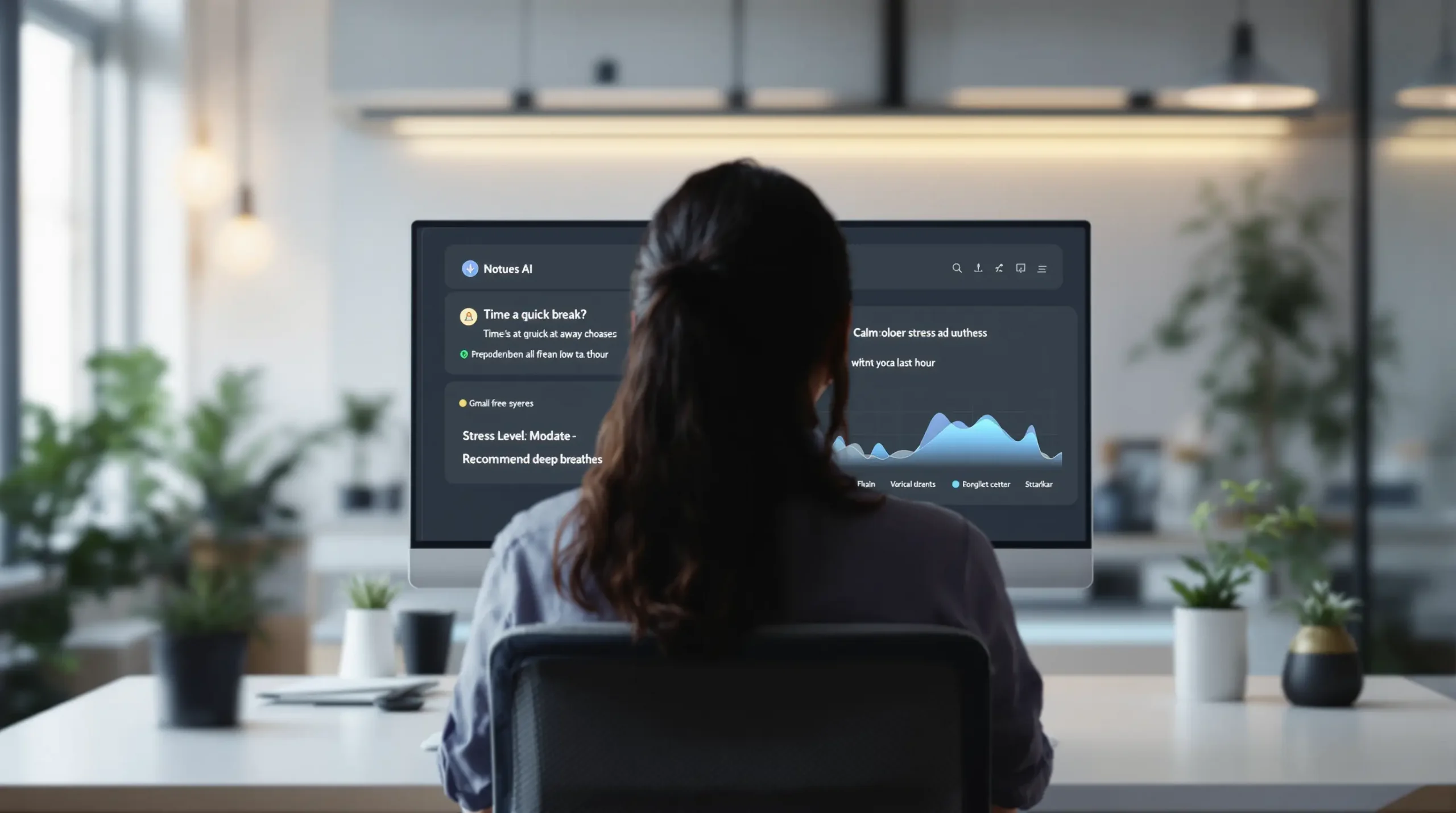
The technology has evolved significantly in recent years, progressing from basic sentiment analysis (positive/negative/neutral) to more nuanced emotional detection that can distinguish between closely related feelings like frustration, disappointment, or anxiety. This advancement represents a crucial step toward truly human-like AI interactions.
The Science Behind Emotion Detection
Emotion recognition AI employs several scientific approaches to understand human feelings:
Text-based Emotion Analysis
Natural Language Processing (NLP) algorithms analyze written text – from chat messages to emails – to identify emotional content. These systems evaluate:
- Word choice and emotional vocabulary
- Syntax and sentence structure
- Punctuation patterns (like excessive exclamation points!!!)
- Use of emoticons and emojis 😊 or 😠
- Contextual cues within the conversation
Modern text analysis models can detect subtle shifts in tone and understand complex emotional states beyond simple positive/negative classifications.
Voice-based Emotion Recognition
When analyzing speech, AI systems examine:
- Pitch variations and intonation patterns
- Speaking rate and rhythm
- Volume and intensity
- Voice quality (breathiness, tension, etc.)
- Pauses and hesitations
These acoustic features often reveal emotional states that might be hidden in the words themselves, making voice analysis particularly valuable for customer service calls.
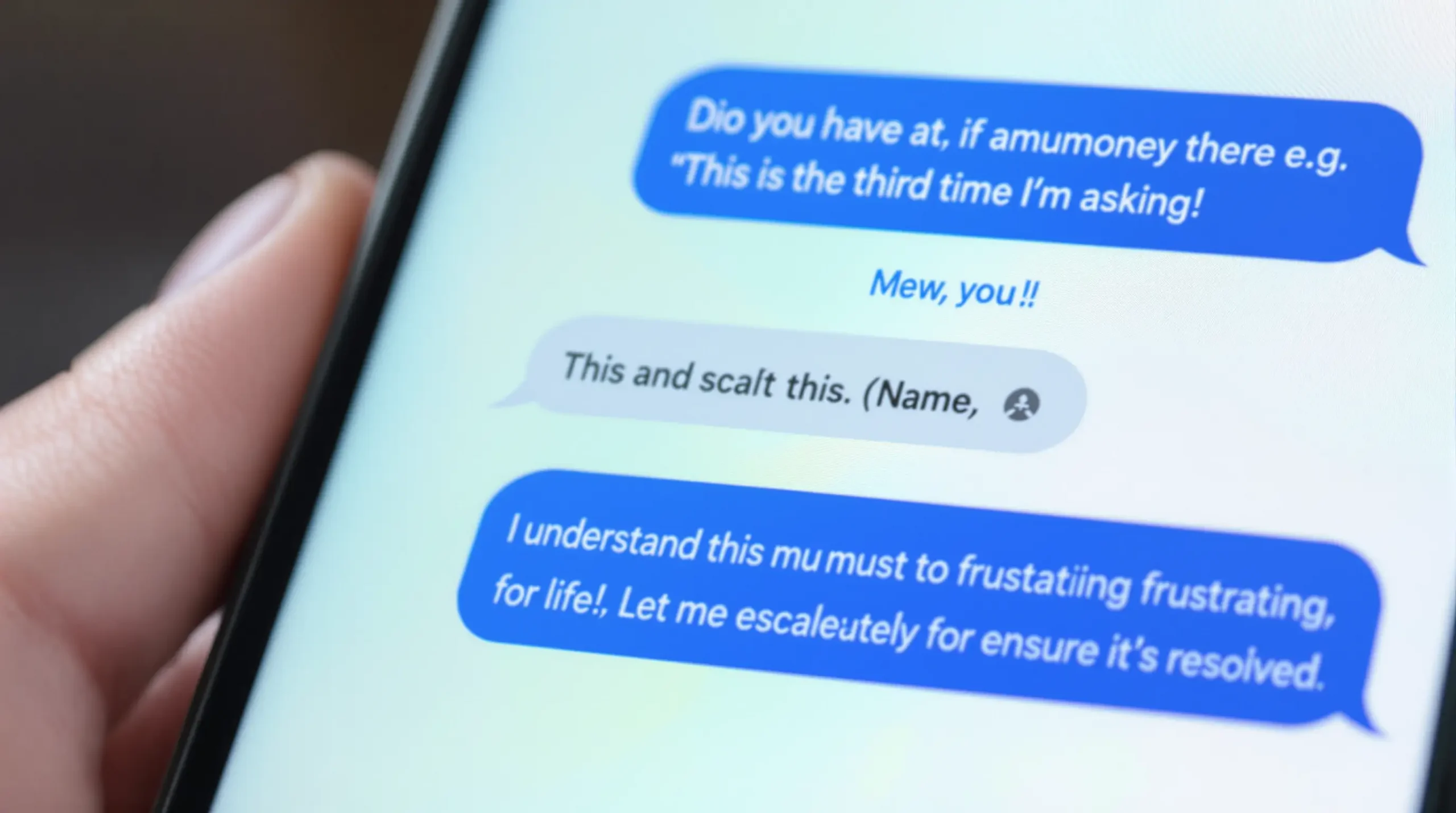
Facial Expression Analysis
For video interactions, computer vision technology analyzes facial expressions by:
- Mapping facial feature points and their movements
- Identifying micro-expressions that indicate emotions
- Tracking eye movements and gaze direction
- Analyzing head position and movement
This approach builds on pioneering research by psychologist Paul Ekman, who identified universal facial expressions tied to specific emotions across cultures.
“The most effective emotion recognition systems don’t rely on a single input channel but combine multiple modalities for a more accurate understanding of how customers truly feel.”
Current Applications in Customer Support
Emotion recognition technology is already transforming customer service operations in several key ways:
Emotionally Responsive Chatbots
Advanced AI chatbots now adapt their responses based on detected customer emotions. When a customer exhibits frustration, the AI might:
- Use more empathetic language
- Offer additional assistance options
- Escalate to a human agent more quickly
- Provide more detailed explanations
This emotional awareness helps prevent the escalation of negative interactions and improves overall customer satisfaction.
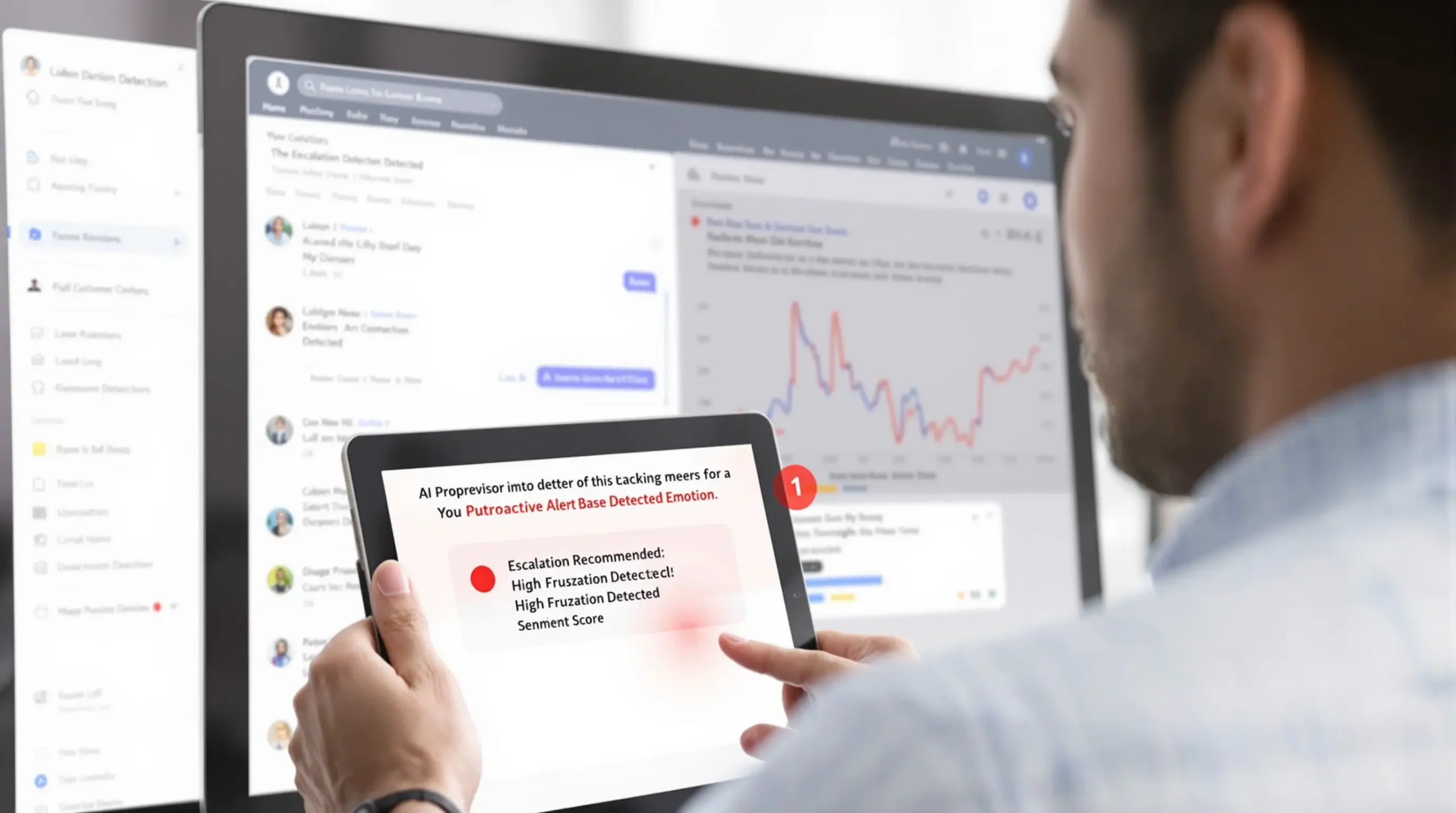
Agent Assistance Systems
Human support agents benefit from real-time emotion analysis through:
- Emotional dashboard displays showing customer sentiment during calls
- Suggested responses based on detected emotions
- Alerts when customer frustration reaches critical levels
- Post-interaction analysis for training purposes
These tools help human agents respond more effectively to emotional cues they might otherwise miss in digital communications.
Customer Experience Analytics
On a broader scale, emotion recognition enables companies to:
- Identify patterns in customer emotional responses to specific products or services
- Pinpoint support issues that consistently trigger negative emotions
- Map the emotional journey throughout the customer lifecycle
- Measure the emotional impact of marketing campaigns and product changes
This data helps businesses make strategic decisions to improve overall customer experience beyond individual interactions.
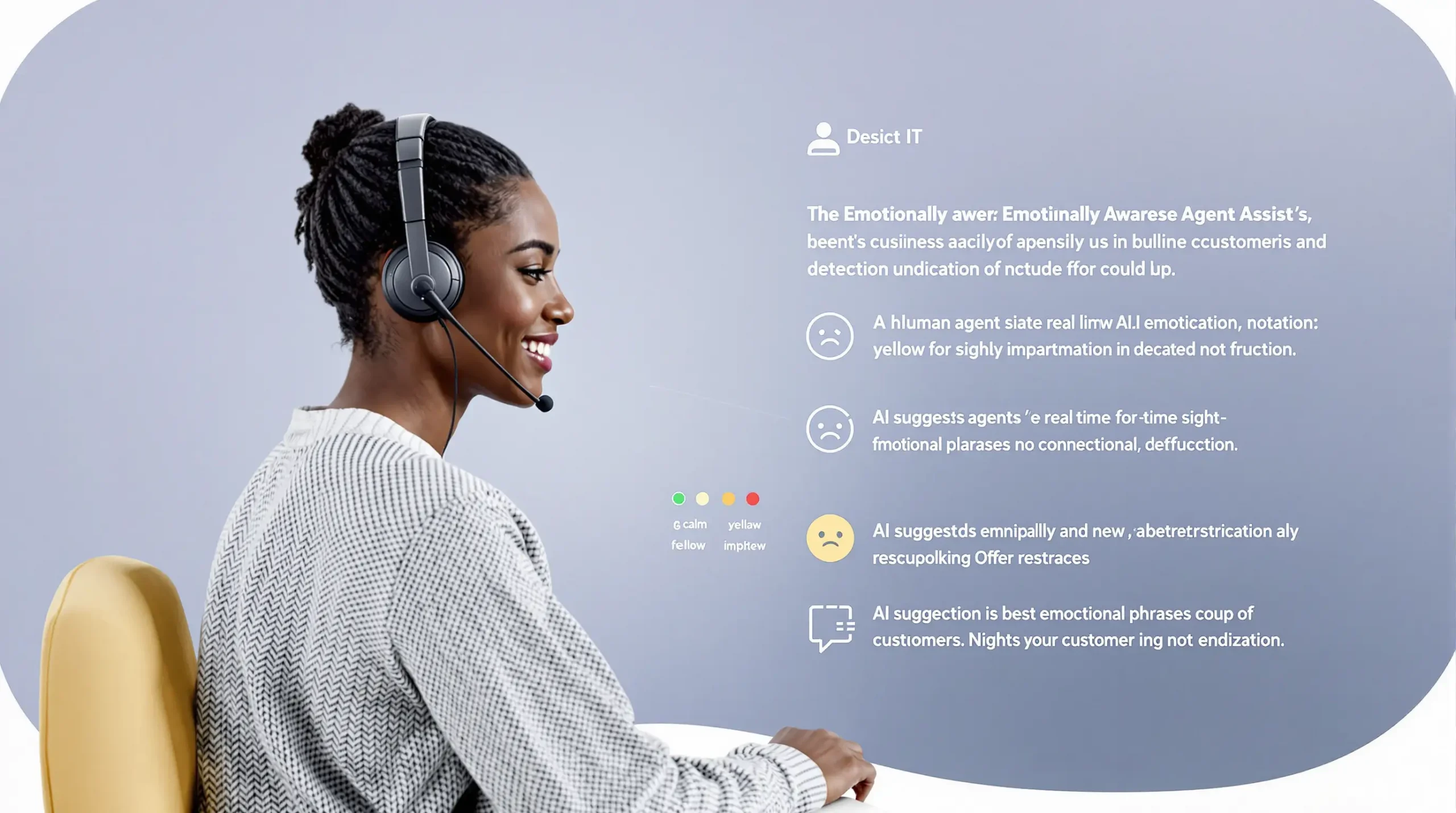
Ethical Considerations and Privacy
While emotion recognition technology offers significant benefits, it also raises important ethical questions:
Consent and Transparency
Customers should be informed when their emotional responses are being analyzed. Best practices include:
- Clear disclosures about emotion detection in privacy policies
- Opt-out options for customers who prefer not to have their emotions analyzed
- Explanations of how emotional data will be used and stored
Transparency builds trust and prevents customers from feeling manipulated or surveilled.
Accuracy and Bias Concerns
Emotion recognition systems can exhibit biases and accuracy issues:
- Cultural differences in emotional expression may not be properly accounted for
- Facial analysis algorithms have historically performed less accurately on certain demographic groups
- Neurodivergent individuals may express emotions in ways that current systems misinterpret
Responsible implementation requires ongoing testing with diverse populations and continuous improvement of algorithms to minimize these issues.
“Ethical emotion AI doesn’t make assumptions about what customers should feel, but rather seeks to understand and appropriately respond to how they actually feel.”
Data Security
Emotional data is inherently sensitive information that requires robust security measures:
- End-to-end encryption for all emotional analysis data
- Clear data retention policies that limit storage duration
- Anonymization protocols that separate emotional profiles from personally identifiable information
- Regular security audits of emotion recognition systems
Protecting this information is not just a legal requirement but an essential component of maintaining customer trust.
The Future of Emotionally Intelligent AI
The evolution of emotion recognition technology is moving in several exciting directions:
Multimodal Emotional Intelligence
Future systems will seamlessly integrate multiple emotional inputs:
- Combining text, voice, and visual analysis for comprehensive emotional understanding
- Tracking emotional patterns over time to build customer emotional profiles
- Contextualizing emotions within the customer journey
- Detecting emotional incongruence between different channels (e.g., positive words but frustrated tone)
This integration will provide a much more nuanced understanding of customer emotions than any single analysis method.
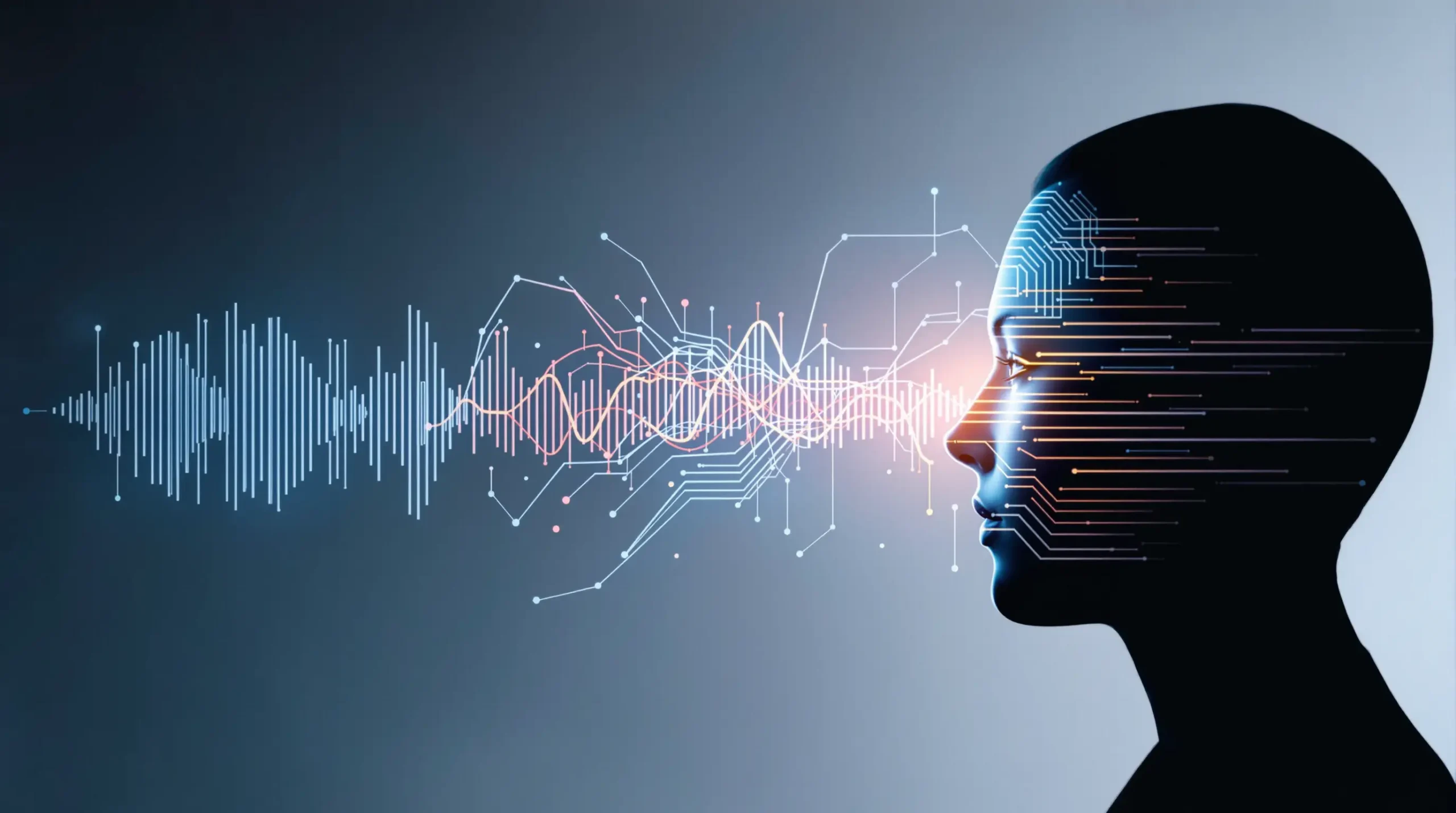
Proactive Emotional Support
Rather than just reacting to detected emotions, advanced systems will anticipate emotional needs:
- Predicting potential emotional responses to specific situations
- Intervening before negative emotions escalate
- Personalizing interactions based on individual emotional patterns
- Offering preemptive support during emotionally challenging moments in the customer journey
This shift from reactive to proactive emotional support represents a significant advancement in customer experience management.
Emotional Regulation and Coaching
The next generation of emotionally intelligent AI may help both customers and support agents:
- Support agents with real-time coaching on emotional intelligence during difficult interactions
- Assist customers with emotional regulation during frustrating experiences
- Provide emotional feedback to improve communication effectiveness
- Create emotionally balanced interactions that benefit all parties
These developments point toward AI becoming not just an emotional detector but an emotional coach that improves human-to-human interactions.
Integrating Emotion AI into Your Business
For companies considering emotion recognition technology, here are key implementation strategies:
Starting Small
Begin with focused applications:
- Text-based sentiment analysis for chat support
- Voice analysis for call center quality monitoring
- Post-interaction surveys with emotional assessment
These entry points provide valuable data while minimizing implementation challenges.
Training and Change Management
Prepare your team for emotionally intelligent systems:
- Train support agents to work alongside emotion AI tools
- Develop protocols for handling emotionally charged customer interactions
- Create guidelines for when to escalate based on emotional indicators
- Establish metrics that balance emotional outcomes with traditional performance indicators
Employee buy-in is essential for successful implementation of emotion recognition technology.
Measuring Success
Evaluate the impact of emotion AI using both traditional and emotional metrics:
- Customer satisfaction and Net Promoter Score changes
- Reduction in escalation rates for negative emotional interactions
- Emotional journey mapping through the customer lifecycle
- Agent confidence and satisfaction when working with emotionally intelligent systems
A comprehensive measurement approach helps justify investment in emotion recognition technology and guides ongoing improvements.
Need expert help with AI customer support for your business? Contact us for tailored solutions. You can also test our AI customer robot developed for Shopify here: Test our AI Chatbot.
Frequently Asked Questions
How accurate is emotion recognition AI currently?
Current emotion recognition AI systems vary in accuracy depending on the technology used and the emotions being detected. Text-based sentiment analysis typically achieves 70-85% accuracy for basic emotional categories. Voice emotion recognition systems generally range from 65-80% accurate, while facial expression analysis can reach 75-90% accuracy for primary emotions in controlled settings. Complex or subtle emotions remain challenging, and accuracy can decrease in real-world environments with background noise, poor lighting, or culturally diverse populations. Multimodal systems that combine different analysis types generally achieve higher accuracy rates than single-mode approaches.
Can emotion AI really understand cultural differences in emotional expression?
This remains a significant challenge for emotion recognition AI. While basic emotions like happiness, sadness, and anger have some universal expressions, cultural norms heavily influence how emotions are expressed and interpreted. Advanced systems are being trained on more diverse datasets and incorporating cultural context into their analysis models. Some platforms now offer culture-specific training for their emotion recognition systems. However, true cross-cultural emotional understanding is still developing, and businesses should be aware of these limitations when implementing emotion recognition globally. The most effective systems incorporate cultural metadata and adapt their interpretation frameworks based on detected cultural contexts.
What are the biggest privacy concerns with emotion recognition technology?
The primary privacy concerns include: 1) Collection of intimate emotional data without clear consent; 2) Potential for emotional manipulation through targeted responses; 3) Creation of emotional profiles that could be used for discriminatory purposes; 4) Cross-contextual use of emotional data beyond its original purpose; 5) Security vulnerabilities that could expose highly sensitive emotional information. Organizations implementing emotion recognition should address these concerns through transparent data policies, strong security measures, clear opt-out mechanisms, and strict limitations on data retention and usage. Numerous jurisdictions are currently developing specific regulations for biometric and emotional data collection that may impact implementation requirements.
How does emotion recognition AI help human support agents?
Emotion recognition AI supports human agents in several key ways: 1) Real-time emotional dashboards alert agents to customer distress that might not be evident in text communications; 2) Suggested responses based on detected emotions help agents respond appropriately; 3) Escalation notifications when emotions reach critical thresholds enable timely intervention; 4) Post-interaction emotional analysis provides training opportunities to improve emotional intelligence; 5) Emotion tracking across a customer’s history gives context for current interactions. These tools work best when used to augment human emotional intelligence rather than replace it, helping agents deliver more empathetic and effective support.
What’s the difference between sentiment analysis and emotion recognition?
Sentiment analysis typically classifies text into positive, negative, or neutral categories, providing a general polarity assessment. Emotion recognition is more nuanced, detecting specific emotional states like joy, anger, fear, surprise, disgust, or sadness. While sentiment analysis focuses primarily on the valence of communication (good vs. bad), emotion recognition aims to identify the actual emotional state behind the communication. Modern emotion recognition systems often go beyond basic emotion categories to detect complex emotional states like confusion, frustration, relief, or interest. This granular emotional understanding enables more tailored responses than simple sentiment polarity.
0 Comments