Rule-Based vs. AI-Powered Customer Support: Key Differences
Estimated Reading Time: 8 minutes
Key Takeaways
- Rule-based systems follow predefined rules and decision trees, while AI-powered solutions use machine learning to understand context and intent
- AI customer support offers superior scalability, personalization, and language understanding capabilities
- Rule-based systems provide consistency and predictable responses but lack adaptability to complex scenarios
- The future of customer support will likely involve hybrid models combining rule-based reliability with AI flexibility
- Businesses should consider their specific needs, customer base complexity, and technical resources when choosing between these approaches
Table of Contents
As businesses strive to improve customer experiences while managing operational costs, the choice between rule-based and AI-powered customer support systems has become increasingly significant. These two approaches represent fundamentally different philosophies in handling customer inquiries, each with distinct advantages and limitations.
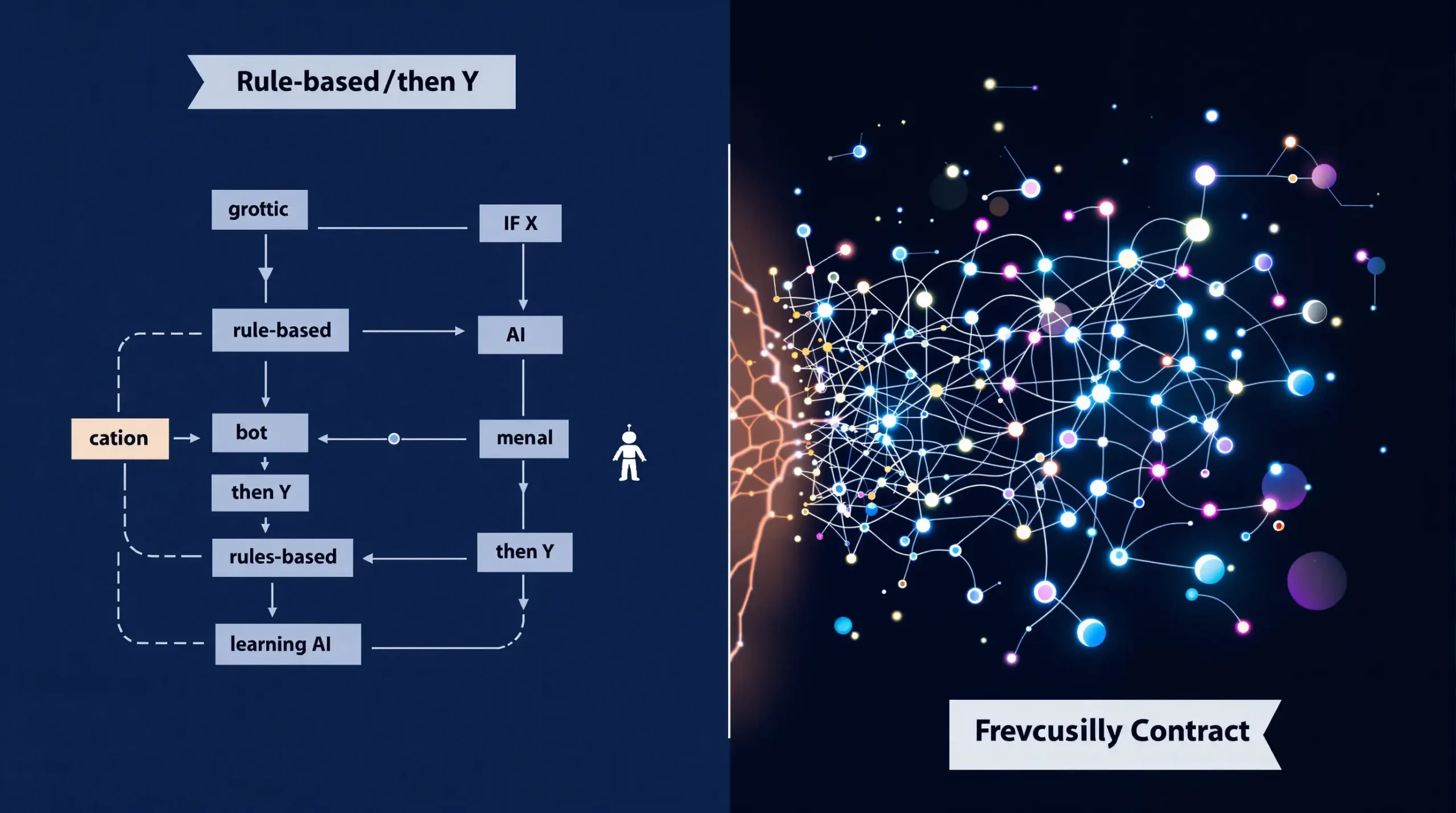
Understanding Rule-Based Systems
Rule-based customer support systems operate on a predetermined set of rules and decision trees. These systems follow an “if-this-then-that” logic pattern, where specific customer inputs trigger corresponding predefined responses.
How Rule-Based Systems Work
Rule-based systems function through a series of conditional statements. For example, if a customer asks about return policies, the system identifies keywords like “return” or “refund” and provides the corresponding pre-written information. These systems typically incorporate:
- Decision trees with multiple branches
- Keyword recognition to categorize inquiries
- Predetermined responses for recognized query patterns
- Escalation protocols when queries fall outside defined rules
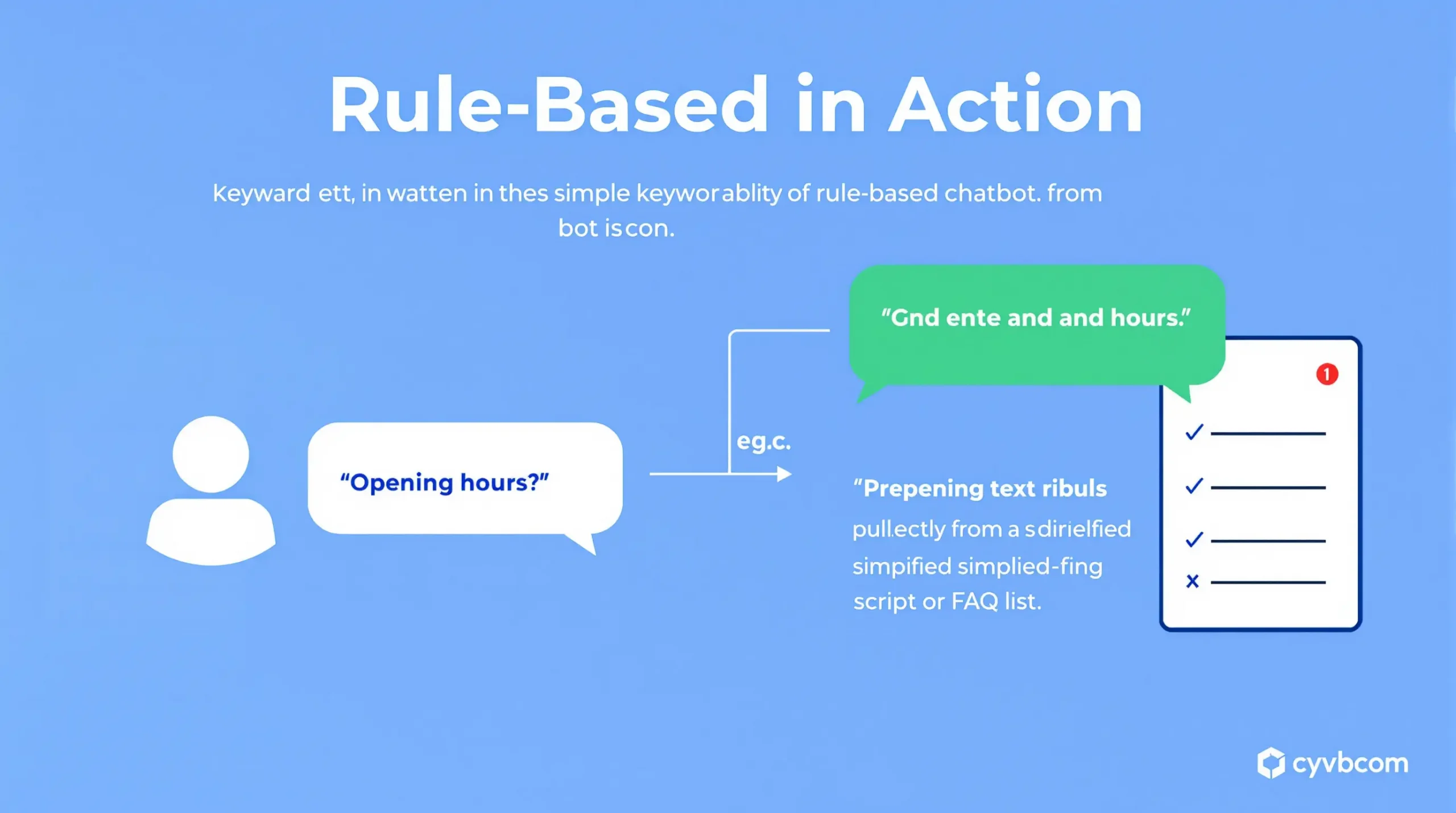
Advantages of Rule-Based Approaches
Rule-based systems offer several distinct benefits:
- Predictability: Responses are consistent and follow established company guidelines
- Simplicity: Easier to implement and maintain without extensive technical expertise
- Transparency: Clear understanding of how decisions are made and responses are generated
- Lower initial cost: Generally less expensive to develop compared to AI solutions
“Rule-based systems excel in environments where customer inquiries follow predictable patterns and where compliance with specific wording is essential.”
AI-Powered Customer Support Explained
AI-powered customer support leverages machine learning, natural language processing (NLP), and often large language models (LLMs) to understand, interpret, and respond to customer inquiries in a more human-like manner.
Core Technologies Behind AI Support
AI customer support systems are built on several sophisticated technologies:
- Natural Language Processing: Enables systems to understand the nuances of human language
- Machine Learning: Allows the system to improve its responses over time based on new data
- Sentiment Analysis: Helps identify customer emotions to provide appropriate responses
- Intent Recognition: Determines what the customer is trying to accomplish beyond just keywords
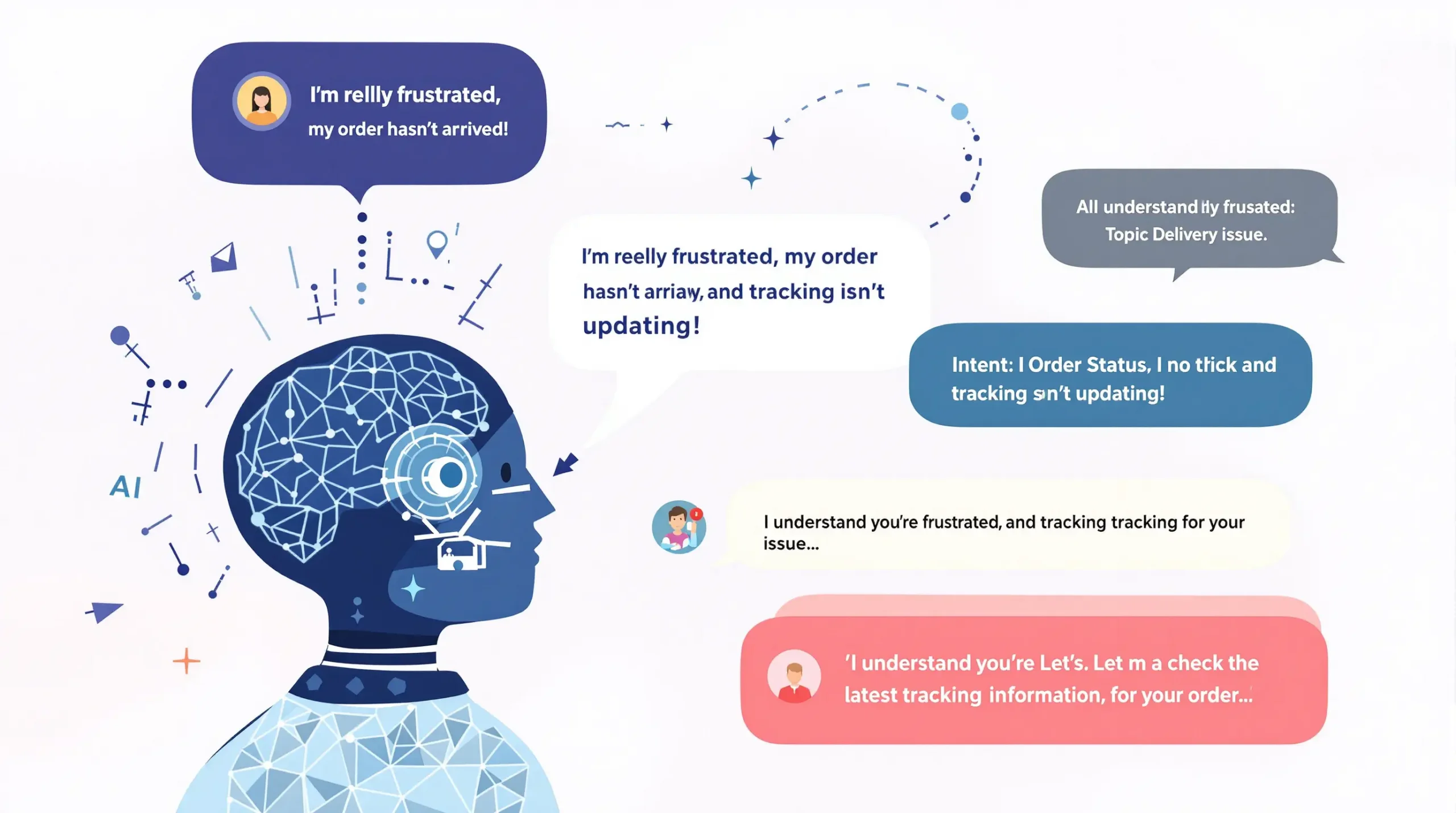
Advantages of AI-Powered Approaches
AI-powered systems offer considerable advantages in complex customer support scenarios:
- Contextual understanding: Comprehends query meaning beyond simple keywords
- Adaptability: Learns from interactions to improve future responses
- Personalization: Can tailor responses based on customer history and preferences
- Language flexibility: Handles diverse phrasings, slang, and even typos effectively
Key Differences in Functionality
The fundamental differences between rule-based and AI-powered systems manifest in several key functional areas.
Understanding and Interpreting Customer Queries
Rule-based systems operate primarily through keyword matching and predefined patterns. While effective for straightforward inquiries, they struggle with:
- Variations in how questions are phrased
- Misspellings or grammatical errors
- Multiple questions within a single inquiry
- Context from previous conversations
AI-powered systems excel at natural language understanding, allowing them to:
- Understand the intent behind diverse phrasings
- Account for conversation history and context
- Handle imperfect language and typos gracefully
- Extract multiple intents from complex inquiries
Response Generation and Quality
The approach to generating responses differs significantly between the two systems:
- Rule-based responses are pre-written and templated, ensuring consistency but often feeling mechanical and generic
- AI-generated responses are dynamically created, potentially leading to more natural, conversational interactions that address the specific nuances of each query
“The difference between rule-based and AI systems is most apparent when customers phrase questions in unexpected ways or present complex scenarios that span multiple support categories.”
Scalability and Adaptability Comparison
As businesses grow and customer needs evolve, the scalability and adaptability of support systems become crucial considerations.
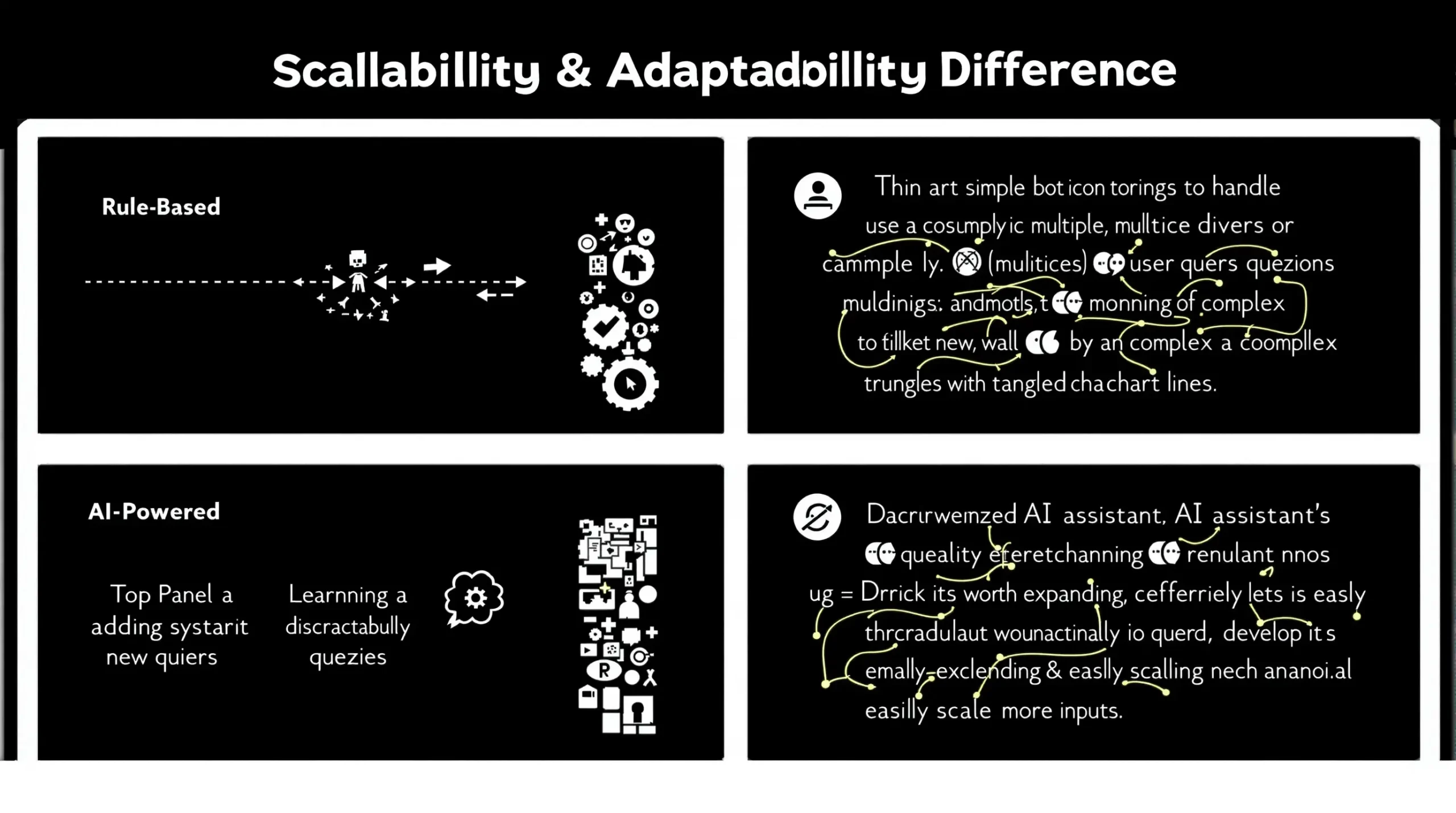
Handling Increasing Volume and Complexity
Rule-based systems face significant challenges when scaling:
- Each new product, policy, or service typically requires new rules
- Decision trees become increasingly complex and difficult to maintain
- Rule conflicts and edge cases multiply as the system expands
- Human intervention is required more frequently as query diversity increases
AI-powered systems demonstrate superior scalability:
- Can be trained on new information without complete restructuring
- Handle increasing query volume without proportional increases in complexity
- Improve performance over time through learning from interactions
- Adapt to changing customer behavior patterns
Adapting to Changing Business Needs
Businesses constantly evolve, and their customer support systems must keep pace:
- Rule-based systems require explicit reprogramming for new offerings, policy changes, or seasonal variations
- AI systems can be updated through additional training data and can often identify new patterns in customer behavior without explicit programming
Implementation Considerations
When choosing between rule-based and AI-powered customer support, several practical considerations come into play.
Resource Requirements
The implementation of these systems requires different resources:
- Rule-based systems:
- Lower initial development costs
- Content writers for response templates
- Business analysts to define logical flows
- Ongoing maintenance to update rules
- AI-powered systems:
- Higher initial investment
- Data scientists for model training
- Substantial training data requirements
- Computational resources for processing
Integration with Existing Systems
Integration capabilities can significantly impact implementation timelines and success:
- Rule-based systems often offer simpler integration with existing customer support infrastructure but may have limited API flexibility
- AI systems typically provide more robust APIs and integration options but may require more sophisticated data connections to operate effectively
Measuring Success and ROI
Success metrics differ somewhat between the two approaches:
- Rule-based metrics often focus on containment rates, correct categorization, and escalation percentages
- AI system metrics typically include accuracy improvements over time, customer satisfaction scores, and resolution rates for complex queries
The Future of Customer Support
The landscape of customer support technology continues to evolve rapidly.
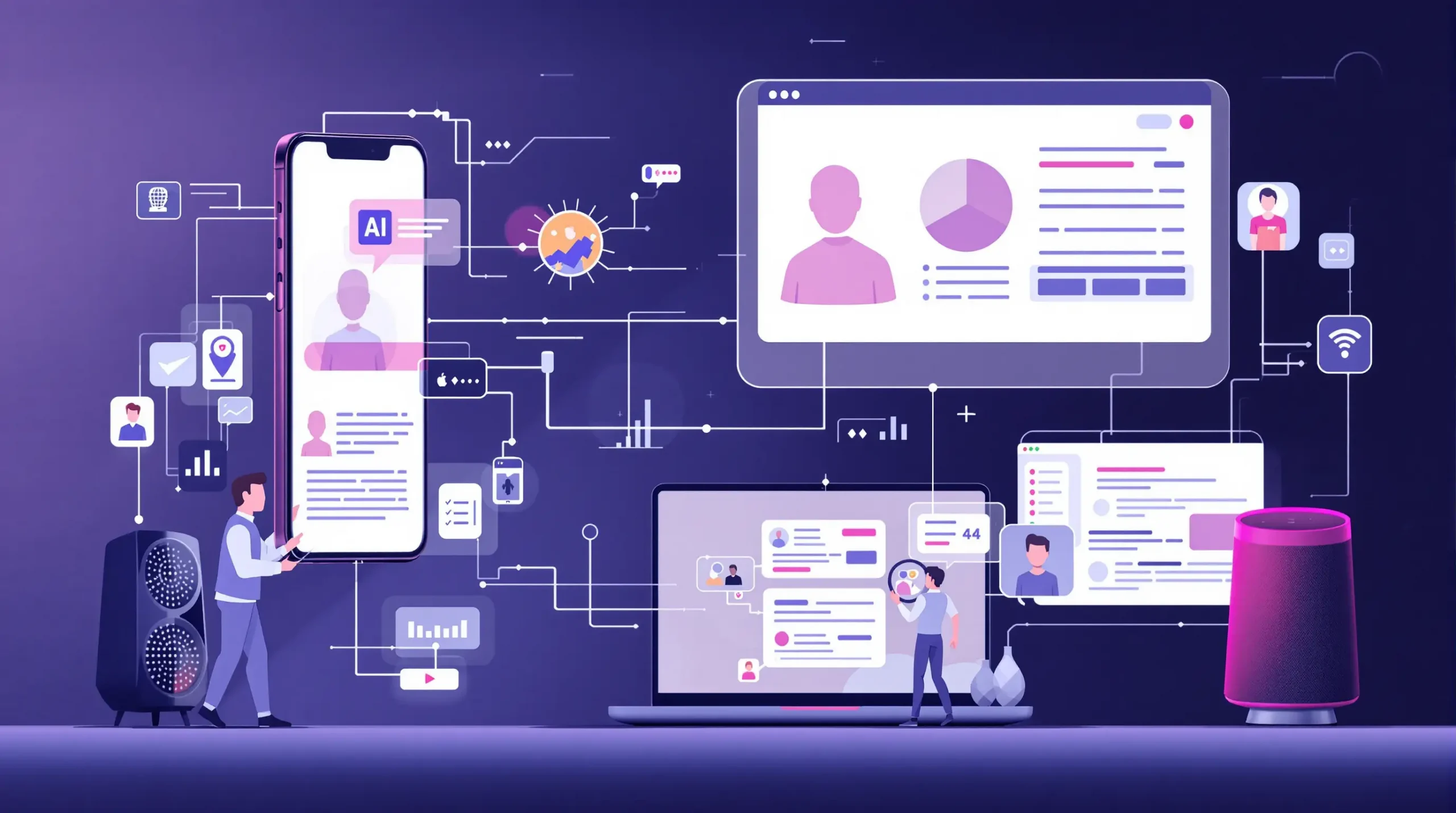
Hybrid Approaches
Many businesses are finding value in hybrid models that combine the strengths of both approaches:
- Using rule-based systems for highly regulated communications where specific language is required
- Implementing AI for complex query understanding and personalization
- Creating seamless handoffs between rule-based processes and AI-powered assistance
Emerging Trends
Several trends are shaping the future of customer support technologies:
- Voice and multimodal AI that can understand and respond through multiple channels
- Emotional intelligence capabilities that recognize and appropriately respond to customer sentiment
- Predictive support that anticipates issues before customers need to ask
- Self-improving systems that continuously optimize without human intervention
“The most successful customer support implementations of the future will likely blend the consistency of rules with the adaptability of AI, all while maintaining the human touch that customers value.”
Need expert help with AI customer support for your business? Contact us for tailored solutions. You can also test our AI customer robot developed for Shopify here: Test our AI Chatbot.
0 Comments